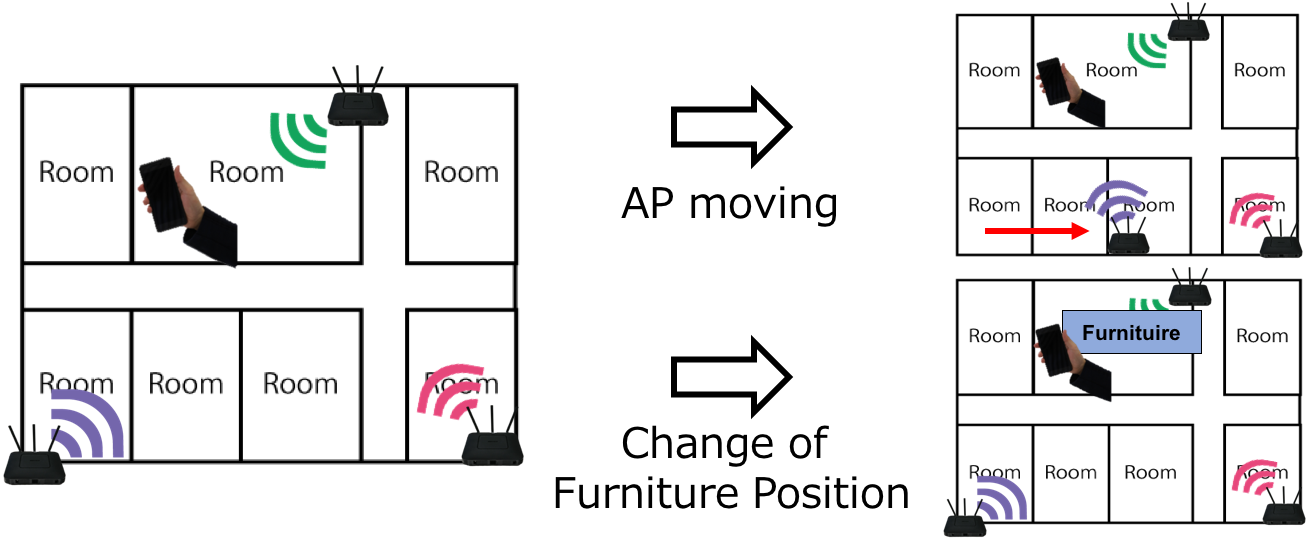
The user’s indoor location is vital information for analyzing user’s activity. RSSI-based localization is prominent technology among the indoor location estimation technologies. However, accuracy deterioration occurs due to furniture or the access point (AP) being moved and other effects in “wild” environments such as shopping malls, and train stations, (i.e., uncontrollable for localization service providers). To recover from such deterioration, data has to be periodically collected and used to retrain the model. A few work on additional data collection and retraining has been explored; however, no work provides way of recovering the accuracy deterioration in a systematic manner.
We propose a new retraining method, called GroupWiLo, to resolve the accuracy deterioration problem. GroupWiLo minimizes the total variation of the parameters, (i.e., parameter changes between the previous term and current term), of the localization models with a new regularization technique. Unlike the traditional approaches (e.g., transfer learning or semi-supervised learning), the proposed method discards the dataset collected for the previous retraining processes. This leads that our retraining process does not increase computational cost independent of the number of retraining.
The experimental results show that GroupWiLo performs as intended and its accuracy is as high as conventional full data retraining methods. Furthermore, GroupWiLo is competitive with the conventional approaches of transfer learning, while its computational cost does not increase.
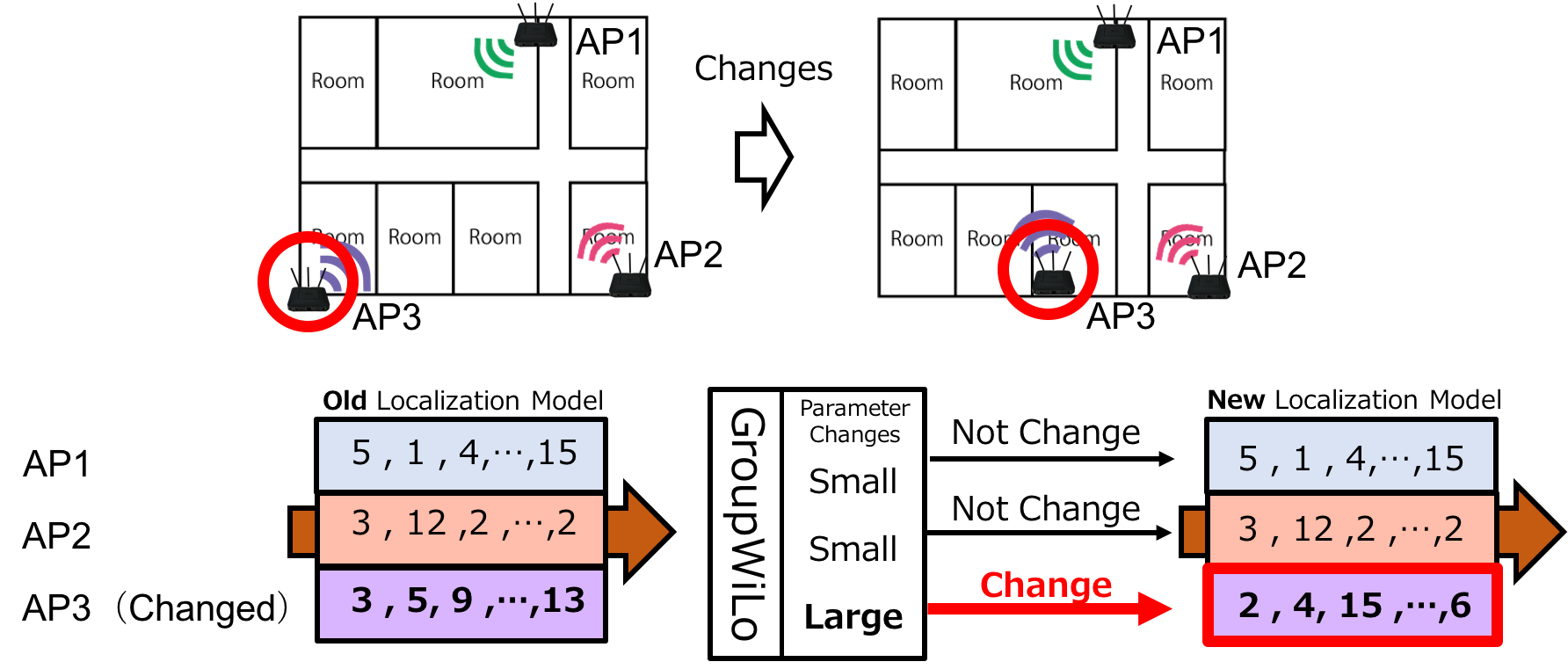
Publications
Masato Sugasaki and Kota Tsubouchi and Masamichi Shimosaka and Nobuhiko Nishio
Group Wi-Lo: Maintaining Wi-Fi-based Indoor Localization Accurate via Group-wise Total Variation Regularization.
2019 International Conference on Indoor Positioning and Indoor Navigation (IPIN).