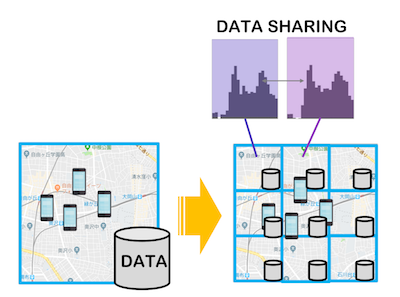
Forecasting the temporal transition of active population in a city, i.e., urban dynamics, is a very important topic from the viewpoint of urban planning, traffic control, and personnel allocation.
We have been pursuing this topic in the vicinity of major stations and sightseeing spots by analyzing a large amount of GPS-based mobility logs, using contextual information such as weather and days of the week [Shimosaka+@UbiComp2015].
The activities of people in a city, as we usually perceive them, have different characteristics for each small area such as the east and west of a station or a shopping street.
Therefore, by dividing the region into fine-grained meshes and forecasting the urban dynamics for each mesh, we can gain a deeper understanding of the nature of the city.
However, since the prediction using the model in [Shimosaka+@UbiComp2015] was based on the location information of only the regions of interest, the amount of data within each region required for training may not be sufficient if such region of interest is divided into fine-grained meshes.
At the same time, as the number of regions to be focused on increased, the number of models and the learning parameters became larger, which resulted in huge learning costs.
Therefore, we proposed a method to solve the problem of insufficient data and the problem of increasing the total number of parameters by representing the forecasting model on each mesh by a mixture of basic models and learning while sharing the data among regions [Shimosaka et al. @SigUbi60][Hayakawa+@PAKDD2021]. Also, it can be assumed that the nature of the active population in neighboring meshes is similar. We incorporated this assumption into a model that shares parameters between meshes [Shimosaka+@AAAI2019].
We confirmed the prediction performance improvement of these proposed models through experiments using GPS mobility logs on a mesh divided into high resolution. Furthermore, additional experiments demonstrate that the mixture model-based approach [Hayakawa+@PAKDD2021] is superior to the parameter-sharing approach among neighboring regions [Shimosaka+@AAAI2019].
Publications
携帯端末位置履歴を用いた階層ディリクレ混合回帰モデルに基づく活動人口予測.
情報処理学会研究報告 第60回UBI合同研究発表会, 兵庫県淡路市, 12 2018.
Masamichi Shimosaka and Yuta Hayakawa and Kota Tsubouchi
Spatiality Preservable Factored Poisson Regression for Large Scale Fine Grained GPS-based Population Analysis.
In Proceedings of the Thirty-Third AAAI Conference on Artificial Intelligence (AAAI-19), Honolulu, Hawaii, USA, February 2019.
Yuta Hayakawa, Kota Tsubouchi, and Masamichi Shimosaka
Simultaneous multiple POI population pattern analysis system with HDP mixture regression,
In Advances in Knowledge Discovery and Data Mining: Proceedings of the 25th Pacific-Asia conference on Advances in Knowledge Discovery and Data Mining (PAKDD-2021), Lecture Notes in Computer Science book series (LNCS), vol. 12712, pp. 791-803, May 2021.