We are developing the machine learning method for estimation about
pedestrian number by using single camera in the crowded environments.
Counting people is valuable for applications such as traffic analysis.
In the crowded environment, detection or tracking of each individual
person is difficult because of occlusion. To resolve this problem, we
employ an approach using multiple holistic image features, such as total
number of area around people. In this approach, the well-designed
combination pattern of features and eliminating the non-useful features
improve the estimation accuracy. Therefore, we propose the learning
method which selects only useful features from a large number of
features. Our method contains L1-norm regularization, which is known as
a shrinkage and selection technique, in the segmentation stage and the
feature extraction stage. Experimental results about our method show
sufficient estimation accuracy and reduction of training time.
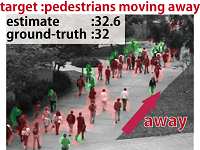